Repsol’s InWell.ai leverages data analytics, AI to enhance drilling, prevent major downtime events
Tool has already boosted ROP by 10-15%; additional development under way to enable remote operations using data-driven decisions
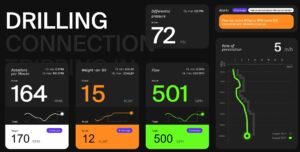
By Javier Rey, Repsol
Data analytics play a vital role in enhancing drilling efficiency, safety and overall operational performance in well construction operations. The integration of AI in the oil and gas industry is a game-changer, offering new perspectives for performance analysis and decision making, ultimately adding value to operations.
Technological advancements now allow high-quality real-time data from rigs and downhole tools to be aggregated and streamed every second, or every few seconds, into visualization tools and cloud databases. Fortunately, big data techniques enable faster processing of large volumes of information, providing valuable insights for proactive performance improvements. This changes the approach to optimize performance, moving from traditional methods reliant on the regional experience of field supervisors and engineers (personnel and behaviors dependent), to new methods that allow the data to speak for itself, thus driving decision making and leading to higher efficiency.
This is the context that motivated Repsol to set an ambitious goal in 2020 for InWell, its Real Time Operations Center (RTOC): becoming a data-driven remote-control center. The initial step toward this goal involved integrating AI for data analytics, which meant the start of InWell.ai.
Development and implementation
The design of this tool was based on three foundational principles:
- Machine learning (ML) for prescriptive analytics: moving beyond post-mortem analysis and forecasting, focusing on providing recommendations for the most efficient course of action.
- Flexibility: adapting to Repsol’s diverse portfolio of operations and to varying wellbore conditions.
- Real-time impact: Enabling immediate adjustments to ongoing operations to maximize performance.
- After months of development, testing and collaboration among field personnel, drilling engineers, data scientists and architects, InWell.ai became operational in Q3 2021. With nearly three years of experience and monitoring over 200 wells, it has improved performance, leading to time and cost savings.
The tool incorporates prescriptive models, combined with physical and statistical models, for four activities: drilling, washing/reaming, tripping and connections. An activity recognition engine, utilizing advanced multivariable analysis with conditional logic, orchestrates these activities by identifying the ongoing operation and activating the corresponding module.
Screens installed in the driller’s cabin display the recommended actions, and modules were designed to provide straightforward and relevant guidance for each activity, simplifying the driller’s tasks and preventing information overload. The end-to-end process, from data ingestion to on-screen display, takes less than five seconds, enabling immediate field action to impact operations in real time.
Drilling Module and machine learning algorithms
ML shows its potential in the Drilling Module, where three algorithms compete to provide the optimum parameters that increase the rate of penetration (ROP) while making hole:
- Offset Well-Based Algorithm: trained with nearby wells’ performance in the current formation. Reference wells are predefined, and the model learns effective levers from their historical data.
- Inter-formation Algorithm: trained with the last 100 m performance in the current well. This model is especially interesting when drilling through transition areas with multiple formations, and it ensures that the optimum parameters in that interval are kept.
- Formation-specific Algorithm: considers the performance observed in the current formation for the well being drilled, having greater impact on long formation intervals, such as in horizontal wells.
The competition among these algorithms is based on the statistical analysis of how each of the models correlates with the performance observed in the previous meters drilled, meaning that the model output is compared with the actual performance to determine which is the algorithm that adapts best to current wellbore conditions. This achieves flexibility, by adapting the model and selecting the best reference parameters for any given interval of the well.
The winning models simulate different scenarios of parameter combinations within an optimization grid to maximize the ROP. This grid is limited by a certain deviation from current parameters, to prevent major changes to any of the drilling levers. Additionally, operational windows are set for each parameter to prevent damage to any bottomhole assembly (BHA) element or to accommodate wellbore condition limitations. These boundaries are also considered within the optimization grid when seeking the optimum ROP.
The last step involves the mechanical specific energy (MSE), considered in the final decision making to determine the combination of parameters. For a similar performance, the MSE involved in the drilling process is minimized to protect downhole tools and maximize on-bottom times.
This complex process, which runs in the cloud in a matter of seconds, ensures that the most suitable parameters are applied. The driller gets the updated recommendation on the screen for weight on bit (WOB), rotation and flow rate, and takes action to improve performance.
Advanced analytics on exploration layer
ML algorithms primarily target development projects due to the abundance of data from offset wells to feed the models. However, exploration wells can also benefit from ML techniques and other advanced analytical tools. The addition of an exploration layer has made the tool more versatile, allowing it to adapt to various scenarios. This layer, built on top of ML algorithms, takes a distinct approach to finding the optimal parameter combination. By starting with a random parameter set, drilling levers are adjusted using AI techniques, like gradient descent or genetic algorithms, until the “sweet spot” is identified. Drillers follow the recommended parameter changes, similar to a drill-off test, to maximize the ROP.
Experience has demonstrated that the exploration layer is beneficial for projects with limited offset well data and during the initial stages of new sections, where parameters are incrementally adjusted. Additionally, this layer proves valuable for projects that maintain steady parameters, allowing testing beyond the comfort zone where the learning capacity of ML algorithms is constrained. Depending on project requirements, the exploration layer can be selectively activated at specific intervals or with a certain frequency.
Impact on flat time operations
The subsequent modules focus on minimizing invisible lost time (ILT) during flat time operations. These modules primarily rely on physical and statistical models, aiming to optimize performance while emphasizing HSE as the top priority. HSE key performance indicators (KPIs) reinforce this message and prevent any loss of focus.
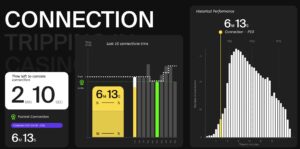
When drillers perform tripping operations for drill pipe or casing, they often ponder several critical questions: Is my borehole clean enough? Should I anticipate restricted intervals or tight spots based on the previous trips? How quickly can I run or pull my BHA to prevent surge and swab? Am I using the correct parameters while reaming or backreaming? This valuable feedback, captured at the rig site, served as the primary reference for designing both the Tripping and Reaming modules.
To address these questions, specific displays were designed to provide comprehensive answers in a single visualization, including the following features:
- Torque and drag engineering simulations compare model curves against actual values to assess borehole cleanliness and identify trends toward higher friction factors.
- Surge and swab simulations at every stand/joint tripped to calculate the maximum speed to prevent downhole issues. Plots comparing current trip performance versus maximum tripping speeds are included to determine the room for potential improvement.
- Advanced analytics for the automatic recording of tight spots. Points are displayed in an interactive plot for upcoming runs, including reaming and backreaming intervals. Drillers have a valuable reference for when they are reaching a problematic area, so they can take precautions and adjust tripping speeds accordingly. Furthermore, this plot includes alerts with relevant information while tripping to enhance communications, such as problematic formation changes, kick-off points, landing points, gas peaks or faults.
The latest module affects drilling and tripping connection times. Dynamic targets adapt to crew performance, offering small incentives to motivate drillers and prioritizing HSE. The module features real-time benchmarks that display drillers’ performance, leveraging statistical rig data, and countdowns to encourage high performance levels.
Prevention of major downtime events
An additional feature impacting drilling and tripping operations was developed to prevent major downtime events, such as stuck pipes. AI techniques help identify major deviations or anomalies that could lead to potential downhole problems. These deviations are contextualized based on current operations, and alerts are prioritized according to severity and associated potential problems. If an alert or alarm is raised, a recommended action guides the driller to ensure corrective measures are taken to mitigate problems.
These actions are linked to Repsol’s internal procedures, thus promoting compliance with regulations. Proper calibration of these models is crucial to receive accurate warnings, and thresholds should be adjusted based on the expected problems to be faced.
Impact and continuous improvement
Over a million meters drilled with InWell.ai in four countries, including onshore and offshore operations, demonstrate that leveraging AI provides significant benefits in terms of time and cost. By enhancing operational performance, Repsol has achieved a substantial impact on ROP, with an overall 10-15% increase when fully complying with or adopting the recommended drilling parameters. The tool has also effectively reduced nonproductive time (NPT), particularly related to stuck-pipe events and hole-cleaning issues.
To unlock the tool’s full potential, continuous model improvement and flexibility to adapt to operations are essential. Rigorous analysis of model outputs validates tool performance, and collaboration between drilling engineers and data scientists is crucial.
One example of this collaboration was the inclusion of the differential pressure of downhole motors in WOB’s and flow rate’s operational window. Engineers identified instances where recommendations exceeded differential pressure limitations, potentially endangering motor integrity. Data scientists adjusted algorithm logic to prevent further increases on these levers once the maximum differential pressure was reached.
Future prospects for InWell.ai
Looking ahead, InWell.ai aims to become the platform for testing remote-controlled operations, applying recommended drilling parameters at rig sites without human intervention. The goal is to automate rotary drilling operations, so that ML model outputs drive operations with data-driven decisions. Drilling automation will also maximize parameter adoption, with a relevant impact on performance based on verified ROP increases from full compliance. This project is under development, and technical feasibility has been confirmed.
Repsol envisions rotary drilling automation like the adaptive cruise control in cars, adapting the speed based on road conditions. In the same way, InWell.ai will adjust the parameters, within the operational window, to maximize the ROP. Just as drivers can regain control of steering and speed, drillers will retain the ability to adjust WOB, rotation and flow rate at any time.
Considering rapid technological evolution, InWell.ai’s future remains wide open. Rather than replacing people with machines, AI will empower drillers to take faster, more efficient actions to enhance performance and safety. Collaborating with other tools and platforms — both internal and from external vendors — will ensure the latest technology is applied, minimizing development times.
At Repsol, the focus remains on realizing data-driven decisions with full support from the RTOC that was envisioned years ago, with data analytics and AI bringing the organization closer to that goal. DC
Click here to watch a video interview with Repsol’s Javier Rey about InWell.ai.