AI and emerging gen AI technologies set to unlock new frontiers in drilling
Industry starting to test the waters with technologies like generative AI to help ‘democratize’ data access, drive smarter decision making
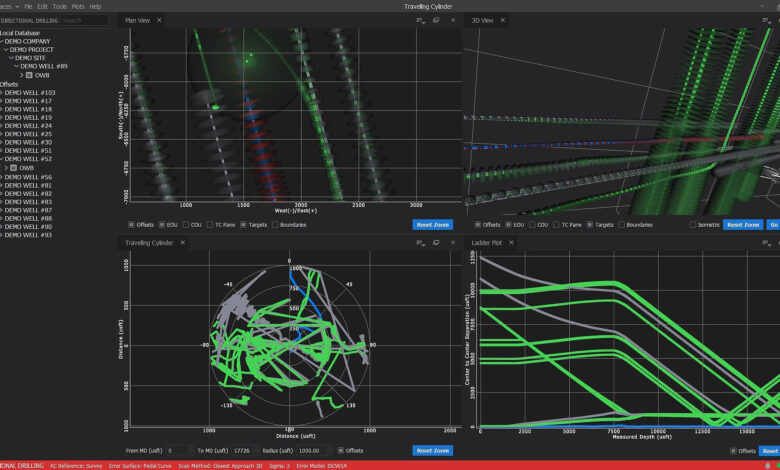
By Stephen Whitfield, Senior Editor
AI and machine learning have become the invisible hands shaping nearly every industry. It is the analytic brain behind facial recognition software, spam filtering and self-driving cars. The oil and gas industry has caught on, deploying new systems that are helping drillers and operators derive powerful insights and predictions based on the mountains of data gathered from rigs, wells and facilities.
Technology developers see the algorithms that fall under the AI umbrella as the key enablers that allow automated systems to optimize drilling operations. They allow companies to drill wells faster while reducing the physical input needed from drillers. For example, an AI-based system can visualize optimal wellbore trajectories for directional wells, saving time in the planning process and removing mundane tasks.
“AI is helping us take all the noise we see with the BHAs, the wells and the formations and taking us to a decision,” said Marat Zaripov, CEO and Founder of AI Driller. “Our goal is to take all that data and process it in a way that helps our clients decide what they should do right now and what their next move should be.”
Even as these AI-based systems are seeing increasing uptake in the drilling industry, AI technology itself is also continuing to evolve at a rapid pace. New systems are emerging based on generative AI (gen AI), which refers to deep-learning models that can take raw data and learn to generate statistically probable outputs when prompted.
Essentially, they encode a simplified representation of their training data and draw from it to create a new work. Generative models have been used for years to analyze numerical data. However, the rise of deep learning has made it possible to extend these models to images, speech and other data types.
“There’s a massive amount of data that’s still being underutilized,” said Jay Shah, Head of Product Marketing for Energy and Utilities at Amazon Web Services (AWS). “We’re looking to simplify the complexity and interactions with data to democratize it. Instead of relying on a pool of analysts to prioritize certain analyses or insights based on certain business workflows, that process can now be in the hands of everyone at all levels of an organization. AI assistance allows you to interact with data. You can simulate processes to help you make decisions.”
A couple of challenges stand in the way of the oil and gas industry widely adopting gen AI, however, according to tech developers who are seeking to deploy their technologies in this industry. First, companies in oil and gas are still seeking the specific high-performing foundational models that can best suit their specific needs. Even if a model has already proven useful in industries like retail and aerospace, they will need to be adapted for oil and gas functions.
Second, companies in oil and gas want to use these foundational models as a base on which they can build differentiated apps using company-specific data, according to developers. Since that data is valuable intellectual property, it must stay completely protected, secure and private during that process. Companies want the integration of these AI systems with their apps to be seamless, without needing to manage huge clusters of infrastructure or incur large costs.
“The companies that we’re talking to are definitely looking to leverage decades of data,” said Brian Land, VP of Global Sales Engineering at Lucidworks, a software company that specializes in commerce and workforce applications. “They’ve been locked into silos – engineering data, seismic data, thermal data – and they want to unlock it. We first had to give people a better way to search for that data. Now it’s a matter of looking into the best ways to build intelligence on top of all that data. These energy companies have big data science departments looking at the data and trying to build models on top of it. I think that’s where we’re going.”
Automating directional drilling
AI Driller, founded in 2019 as a startup company, offers a rig automation platform called AI Cloud that uses well data stored on a cloud server for analytics and monitoring of drilling activity. It is equipped with AI- and machine learning-based drilling data processing technology and real-time automation of conventional auto-drillers. This allows engineers to get historical drilling data so they can deliver effective results and drive consistency.
“A lot of discussion around data is ‘garbage in, garbage out,’ ” said Nasikul Islam, Director of Engineering at AI Driller. “If you don’t have a very good foundation of data quality, a lot of those predictive systems won’t work. AI Driller is really about automation and intelligence. We’re automating a lot of the work for the drilling engineers – all those mundane, repetitive tasks. So, the physics-based modeling is very important for the engineers. But what the wellbore tells you and how the downhole data talks to you is equally as important. You have to listen to the wellbore in order to build these models.”
AI Cloud hosts software programs for managing mud motors (AI Motors); early detection of anti-collision risk, washouts and motor stalls (AI Alerts); and a suite of applications for rotary and sliding automation (AI Assistants). The newest addition to the platform is AI Spaces, a desktop software that handles directional planning, reporting, torque and drag, and hydraulics modeling.
The program, launched last year, uses physics-based models calibrated with a user’s historical well data to help predict the optimal trajectory for new wells. Essentially, whenever a company seeks to plan a new well in a given basin, the models built into the program can help automate projections by using the company’s historical data as a basis – it can look at data from wells drilled in similar conditions to automate projections of the new well. The program provides a visual 3D representation of these projections and target lines.
“We are effectively training the models on historical data and calibrating them,” Mr Zaripov said. “When you match the historical data with the new wellpath, the model can make assumptions. If my model is calibrated, I can go ahead and start looking. If I want to do a three-mile lateral in a given basin, I can look at all my data on that basin, get the assumptions from the model and start planning my new well. In doing this, we’re using our tools and our AI methods to perform the normal day-to-day processes of planning the well that people would have done manually before.”
Users can also map the planned wellbore with existing wells drilled on the same pad to minimize collision risk. “We’re automatically sniffing out collision risk. This is something that used to take 20 minutes to calculate manually – we can do it in a second. That’s more time that we’re saving, by using all the processes that go into ensuring separation are all automated. If I’m the rig manager, this is a great time saving,” Mr Zaripov said.
After calculating the optimal wellpath in AI Spaces, users can then leverage the rest of the AI Cloud platform to ensure that the path is executed efficiently, Mr Islam said. The Rotary Assistant module uses machine learning algorithms to automate auto-driller setpoints like weight on bit and rate of penetration. The AI Alerts module uses predictive AI models and sensor data to notify drilling teams of potential downhole dysfunctions, such as stuck pipe and excessive downhole vibration, and automates an alert to the drilling team. This can help to mitigate potential incidents before they become a problem.
By automating these tasks, directional drilling personnel can make more effective use of their time, Mr Islam said. “Companies can design the well in Spaces and create the drilling program, then send it off to the rig for the execution. Every well can have an optimized roadmap to drill 3,000 feet each day, and we need to help the engineers perform that task. With these tools, we can actually drive the operator or the directional driller to focus on validating and verifying the decisions being made,” he explained.
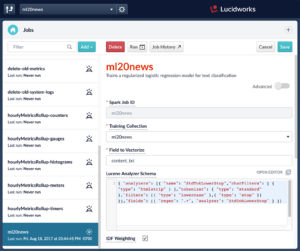
Maximizing data management
While en AI technologies are widely still in the proof-of-concept stage, some companies are beginning to launch systems for commercial use. Lucidworks, for example, has offered machine learning models to multiple industries since 2007 and recently added integration capabilities for gen AI and large language models into its Fusion AI platform.
Mr Land described it as a platform that “grounds large language models in the truth.” It uses existing machine learning models and workflows to index and store records from any data source. It also utilizes Apache Solr, an open-source search engine, and Apache Spark, an open-source analytics engine for large-scale data processing, to process thousands of queries per second from thousands of concurrent users. Essentially, it allows anyone within an organization to search for any piece of data in an instant.
While the gen AI functionality is already being used in other industries, it has not been deployed in oil and gas yet: “Our customers in finance, healthcare and retail have been using it, but with energy, they’re just now talking about it. They want to really test it to make sure it’s accurate, secure, private. With gen AI, you’re dealing with public models, so they really want to make sure there’s no data leakage. But I think in the next year or two, we’re going to start seeing more proof-of-concept work in energy,” Mr Land said.
He believes the benefits that a gen AI platform can provide to oil and gas are clear, however. Users can leverage the technology as a data storage and data access platform, helping employees find information needed to solve problems with specific equipment on a rig or well site.
More specifically to oil and gas, gen AI can help E&Ps optimize field development, especially when it comes to the primary objective of identifying and modeling the hydrocarbon resources that can be exploited cost effectively. When geophysicists work to analyze geology and identify locations to drill, they often run into the challenge of accessing the right information they need. That information resides in a variety of locations and file formats – for example, text files, well logs, GIS files and photos.
For large E&P companies whose operations span decades, internal databases often consist of highly disparate, siloed and unorganized data. This makes it hard for engineers and geophysicists to find and access the right information using conventional search applications. Among the common issues companies face are poor search functionality – available, relevant data take too long for users to access – and inconsistencies in how data are labeled and tagged. Additionally, the information and data related to past projects can sometimes reside solely in the heads of experienced personnel and be inaccessible to new employees.
Gen AI platforms can enable exploration teams to more effectively leverage all the data that are available by giving them the ability to quickly and accurately retrieve information when they need it. This can lead to better decision making.
Fusion collects documents to be processed while maintaining information security and optical character recognition, a process that converts an image of text into a machine-readable format. It can analyze documents via natural language processing and classify relevancy based on the document’s content.
“With upstream, we need to find the best ways to find information like seismic data, well reports and engineering reports that are helping companies make what can be multi-billion-dollar decisions,” Mr Land said. “We’re ingesting lots of big data, like 50-gigabyte seismic files, into Fusion, to make them easier for upstream engineers to find.”
One of the issues with large language models, however, is they’re only as good as the prompts used to elicit a response. There is still a level of variability in the information these models can output, Mr Land said. So, depending on the prompt, users may not realize a desired outcome when using a gen AI system on its own.
To remove this variability, Lucidworks built a library of chained prompts with defined purposes, which can then be selected and deployed by users with minor enhancements. Prompt chaining is a natural language processing technique in which a sequence of prompts is provided to a natural language processing model, guiding it to produce a desired response.
In addition to removing variability, these prompts – known as “guardrails” – can help standardize user scenarios, allowing companies to govern and constrain end-user interactions. For example, the boundary of responses can be set for a certain question – if someone is asking about the geology of a field, the guardrails can help limit responses to discussing only that field.
“Some of the key fears we’ve heard from CIOs are, ‘I don’t want an incorrect answer when I enter a query,’ or ‘I don’t want to show competitive data on a public network that’s not part of my enterprise database,’ or ‘If I’m asking a question, I only want the responses to be about the specific documents I’m asking about.’ While models like ChatGPT are open, we need systems that can constrain it,” Mr Land said.
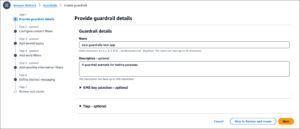
Gen AI in energy
Gen AI is the technology that is leading recent tech innovations like Amazon Bedrock, launched last year. More than 10,000 organizations use the service across multiple industries, including pharmaceutical, automotive and manufacturing, to enable easier searching of historical and real-time data. And now, the platform is finding its way into the energy space.
The cloud service gives companies the ability to access a selection of foundational models from other AI companies (or from Amazon itself) through an application programming interface. Users can experiment, evaluate and apply their data securely to build their own generative AI applications.
Foundational models are large machine learning models that are pre-trained on vast amounts of data. To customize these models, Amazon Bedrock uses retrieval-augmented generation, a technique in which the foundational model connects to additional knowledge sources that it can reference to cull more accurate responses when users search for specific data.
Mr Shah described Bedrock as Amazon’s effort to democratize access to gen AI technologies and simplify the development of gen AI applications – because building and training a foundational model from scratch can be a significantly cumbersome, time-consuming and costly endeavor. The Bedrock software allows companies to use various foundational models as a base for building their own native AI application without the need to train staff on coding, or to manage the associated infrastructure of an AI model.
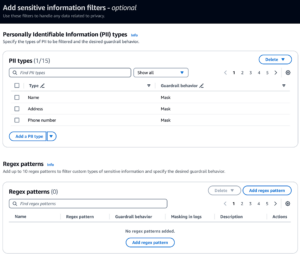
“There’s no one model that addresses everything an organization needs,” Mr Shah said. “You’ll need model choice and flexibility. What Bedrock really provides is a capability to deploy different models for different use cases and for different constraints within an organization – like cost, speed, latency. You’re providing that foundation to where you can develop and deploy different models for different use cases.”
Amazon Bedrock is not currently being used by a drilling contractor, although Mr Shah indicated that AWS is in conversation with drilling contractors about possibly starting pilot programs within the next year. However, the systems have already shown their value in improving safety within oil and gas. CEPSA, a Spanish energy company with operations offshore Algeria and Peru, has been using AWS as its cloud computing provider since 2018.
Bedrock was also used to build the Smart Safety Assistant for CEPSA. Smart Safety Assistant uses historical incident and near-miss reports to improve worker safety. Historically, past incidents and previously known risks are often not considered when issuing work orders for field technicians. But now, supervisors can leverage gen AI to prepare their work orders by summarizing incidents relevant to the current work order tasks.
In 2023, CEPSA began using Smart Safety Assistant in conjunction with Bedrock to help improve safety reporting. Whenever the company had a safety incident at one of its facilities, safety personnel typically received general information related to the incident – where the incident took place or what equipment was involved.
“We wanted to develop packages designed for specific facilities in specific fields,” Mr Shah said. “We want to use generative AI to create a solution that takes weather data, historical incident data, and any additional variable conditions outside, as well as evaluations of near-misses, and contextualizes them with similar things that have happened before. It’s like a personalized briefing, with as much real-time data as is available and as much historical context as is available.”
Through Bedrock, AWS was able to process real-time data from sensors on any equipment involved in a safety incident, as well as weather conditions and near-misses involving those specific pieces of equipment. The result was a more granular report, created within Smart Safety Assistant, that could be used to better inform safety personnel.
Mr Shah said that this functionality provided greater context for safety personnel to address incidents.
“With Bedrock and Smart Safety Assistant, CEPSA was able to take historical incidents and near-miss reports that were in different data silos to improve field worker safety by consolidating that data through generative AI,” he explained. “The incidents that were historically known risks that weren’t used when issuing work orders are now something we can use. That helped the supervisor prepare and contextualize work orders by summarizing incidents relevant to the current work order and tasks that they would look at. What were the near-misses in the past? What were the particular conditions? You’re integrating IoT data and contextualizing them into a safety package.” DC