Integrated platform aims to improve real-time decision making in frac operations
Technology gives engineers actionable insights for optimization while aiming for balance between frac efficiency and effectiveness
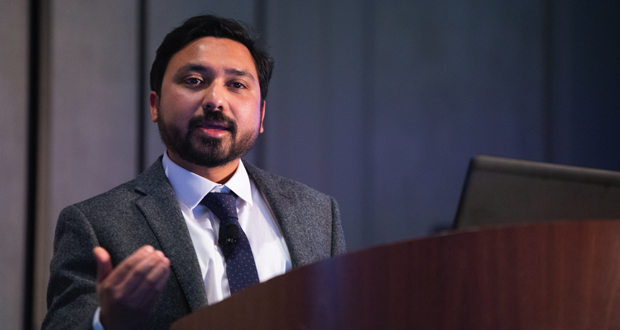
By Stephen Whitfield, Associate Editor
Recent breakthroughs in connectivity and digital technologies are enabling the monitoring and analyses of hydraulic fracturing operations in real time through data streaming and analytics. While most third-party frac monitoring solutions offer things like real-time frac treatment data charts, post-stage frac analytics and key operational efficiency metrics, there was still a need for software that could identify opportunities for frac optimization in real time, augmenting and improving real-time decision making at the frac site.
At the 2022 SPE Hydraulic Fracturing Technology Conference in The Woodlands, Texas, on 2 February, Shell presented a software platform it developed to host and execute an ensemble of third-party frac models and visualizations. It communicates actionable insights within minutes of identifying a potential event during a frac stage. The platform is a plug-and-play system, where users can insert and remove third-party models measuring different variables at a frac site, said Somnath Mondal, Research Production Technologist at Shell.
“Everyone wants to pump the best frac that we can at every stage, but a good frac is not just about pumping away a given volume of water or sand at the lowest cost, but also to get effective stimulation distribution. We’re trying to get a balance of efficiency and effectiveness at every stage,” Dr Mondal said. “We’ve made a tremendous amount of progress in getting real-time data acquisition and running analytics on it, but most market solutions are still not there.”
The platform is based on a fairly simple framework: Real-time sensor data is fed into software for processing and then transferred into third-party models for visualization. To limit the number of variables during lab testing and initial field testing, only models for measuring injection rate and sand concentration were used. However, Dr Mondal said multiple models can be executed simultaneously within the platform. Users can even establish a hierarchical optimization for the whole system based on priority.
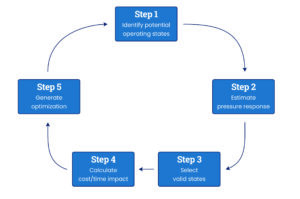
The platform identifies potential operating states for optimization by taking the output from the third-party models and running it through a separate model designed to predict a given action’s impact on pump pressure. This model looks for points where sufficient pressure headroom is available and the pressure trend is favorable, to ensure that any action taken would not increase the pressure outside an acceptable limit for the well. It then identifies the valid operating states that can result from a given change to different variables, and assigns a score.
Another model utilizes historical data to estimate an action’s impact on the completion time and simulates a stage under the assumption of pre-determined control variables. From that simulation, the platform can estimate the cost for each possible operating state and recommend an operating state that is most optimal.
The platform’s recommendations are stored in a data lake, and notifications are sent to engineers about potential events. The recommended actions are presented in the form of a “card” that can be displayed on the user interface. This card includes the suggested change, as well as contextual information to assist engineers in decision making, such as average pressure, predicted pressure and estimated savings. While he did not provide exact figures, Dr Mondal said the estimated savings is typically in the thousands of dollars per recommendation.
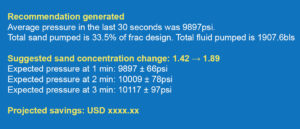
engineers in real-time decision making.
Additional details about the system are available in SPE 209127, “Efficiency and Effectiveness – A Fine Balance: An Integrated System to Improve Decisions in Real-Time Hydraulic Fracturing Operations.”
The platform restricts the frequency of recommendations given within a particular time frame, both to ensure engineers have time to implement the recommendations already given and to see the response to any optimization action taken before a new one is generated.
Dr Mondal stressed that the model is purely a recommendation tool. Engineers at the frac site must decide whether to implement the optimization action. “The idea here is not to replace the human. It’s to provide the human making the decision with the tools they need and the data they need to make the best decision without having to rely solely on experience or recall at a given moment,” he said.
System limitations
While specific results from initial field testing were not discussed, Dr Mondal said the software has consistently identified optimization opportunities and augmented decision making that led to cost savings while preserving frac effectiveness. However, there are limitations. Frequently, the team saw scenarios during testing where the pressure became negatively correlated to the proppant or showed non-linear dynamics due to wellbore complexity. Since the pressure response model used in testing was a linear model, the system’s ability to predict effectively became limited under those scenarios. When the team increased the prediction time frame from 1 minute to 3 minutes during these scenarios, the model became insufficient at modeling the complexities of the system.
To help remedy this issue, the team explored the possibility of incorporating a gradient boosting, or “learning tree,” model into the pressure response. In a gradient boosting model, a base model trains additional models sequentially to try to reduce error. This model leverages historical data and contextual data, such as stage depth. Shell structured the model to input all variables available at a given time and contextual information about the well and stage, such as the depth or formation type.
This model showed impressive predictive power, Dr Mondal said, as it was able to predict the pressure response up to 3 minutes ahead of an event with reasonable accuracy. However, like with the linear model, this model struggled to accurately predict an event in the early ramp phase of the frac stage because there was too much variability.
Shell is currently investigating how to integrate a gradient boosting model into the software platform, which is a challenging task because a large gradient boosting model requires hosting a sizeable number of parameters associated with it. A host would also need to be developed that can cover all possible well types, which is an exhaustive process.
“This model is computationally very heavy,” Dr Mondal said. “You have to keep an evergreen training set, which is very time consuming, and this set needs to constantly update the model. We are trying to keep a balance between the practical implementation challenges and having optimal model accuracy.” DC