Designing for human performance is key to ensure AI deployments are safe, secure and trustworthy
Newly adopted EU AI Act will call for systematic identification, management of risks in applying AI in drilling, as well as better data governance
By Kristian S. Teigen and Fredrik S. Dørum, Norwegian Ocean Industry Authority – Havtil
Did you know that 90% of the world’s data was created in the last two years? And that every other year, until now, the total volume of available data has doubled? In an era where many consider data as the new oil, this exponential increase in data presents both prospects and hurdles.
The race for creating opportunities through leveraging AI for analytics, deriving patterns, trends and insights is well on. However, as is a well-known truth in the drilling industry, most opportunities do not come without challenges. While the utilization of AI for more applications is increasing, both in our workday and for personal performance, the initial awe at AI’s capabilities is increasingly tempered by the realization that these systems also have their limitations and weaknesses.
The weaknesses may stem from a range of sources, varying from how the models are trained, which data (and the quality thereof) they are trained on, the life cycle management and governance of the models, as well as co-dependencies in the digital ecosystem where they operate. As more and more of the data utilized for training AI will be derived from AI itself, there is also a risk of compounding effects that may further amplify bias, inaccuracies and inefficiencies.
The context matters
Having said that, technology development is rarely only about technology. The contexts in which AI is applied matters. Regardless of industry or field of application, a big part of the context relies on our organizational and social frameworks. As a result, all implemented AI solutions become intertwined with social structures, organizational practices, work processes and employee competence.
Even though AI solutions often produce precise results, the reasoning they use is often highly complex and obscure. This can make it difficult for people to understand what the system is trying to optimize for and achieve.
In a recent article, Dr Mica R. Endsley, former Chief Scientist of the US Air Force, points out that Bainbridge’s original “Ironies of Automation” is highly relevant with regards to today’s new wave of AI-enhanced automation. Near-misses and incidents involving human automation operations often arise from a mismatch between the properties of the system as a whole and the characteristics of human information processing.
She also argues that AI, by its very nature, introduces new challenges, such as AI systems being opaque, not open to inspection and AI model drift. Shortcomings in producing accurate and current mental models of the AI, therefore, significantly constrain human ability to anticipate and comprehend AI actions. This limitation diminishes humans’ capacity to respond with appropriate and effective management strategies when a critical situation arises, or if the AI reaches near or outside its operational envelope.
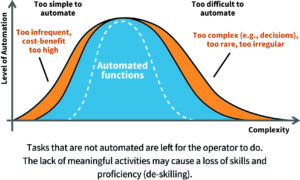
Ensuring meaningful human control
AI and automation involve categorizing tasks into those that can be automated (or left to AI) and those that cannot. This approach bears strong similarity to the approach to mechanization in the previous industrial revolution – “mechanize everything that can be mechanized.” Mechanization increased efficiency and reduced human physical strain, but the trade-off was that the operator was left with whatever (scattered) tasks that were not feasible to mechanize.
This is referred to as the Leftover Principle. It was often considered, including by Alphonse Chapanis – whom many consider the godfather of human factors engineering – to be good practice. The trade-off between leaving the operator with fewer and more scattered leftover tasks in exchange for less physical strain was mutually beneficial.
In their book “Joint Cognitive Systems: Foundations of Cognitive Systems Engineering,” David Woods and Erik Hollnagel argue that, when applied to automation, the Leftover Principle “implies a rather cavalier view of humans since it fails to include any explicit assumptions about their capabilities or limitations.”
Applying the Leftover Principle to AI and automation, the leftover results in humans being assigned two types of tasks that, in many cases, are not well suited for supporting meaningful human control.
The first type includes infrequent tasks, or tasks that are uneconomic to automate. Examples include manual setting and adjustments to offset or to compensate for lack of integration between systems, or performing simple and manual tasks where the cost of automation outweighs the performance benefit.
The second type includes tasks that are too complex, rare or irregular to automate. These may include handling the consequence of unexpected drilling conditions or equipment reliability issues that are difficult for designers to anticipate or automate.
The operator bears responsibility for the far ends of the spectrum of tasks, leaving the operator with an array of tasks that neither constitutes a meaningful set of tasks (which in itself is problematic) but also does not form a sufficient basis for maintaining mental models, skills and situational awareness to resolve the complex task of assuming control at any one time.
Therefore, a balanced approach requires the system design, and the system in itself (to the degree possible), to consider human capabilities and limitations when allocating tasks; it does not assume humans are infinitely adaptable.
Given the dynamic nature of the tasks pertaining to drilling automation, reducing the need for infinite human adaptability would ideally require the system to not only handle a dynamic task allocation between system and driller but also provide it.
With AI enhancement of automation, it is also important that human interaction and meaningful control is supported by transparency, where the system communicates uncertainties relevant for maintaining good situational awareness.
Preparing for the EU AI Act
The newly adopted EU AI Act is founded on a risk-based approach to regulation, classifying systems into risk categories (unacceptable, high, limited and minimal/no risk). The classification of a system largely depends on the context in which the AI is used, as well as the potential impact on an individual and societal level, rather than the complexity or simplicity of the technology. Therefore, the majority of stipulations within the AI regulation are directed toward systems deemed as having high-risk contextual implications.
The span of technologies, models and solutions that fall under the EU AI Act’s scope is wide – from deep learning to machine learning techniques to statistical models and expert systems providing decision support. Whether the AI technology is “wide” or “narrow” is less important for the classification.
In the offshore industry and energy sector, it’s expected that the implementation of AI that can impact the safety of personnel or the integrity of the asset will be considered a high-risk application. Energy production, transportation and distribution fall under the EU AI Act’s definition of critical infrastructure, both directly and through the definition of critical infrastructure set out in other directives, such as the Critical Entities Resilience Act and NIS 2.
Use of high-risk AI will mandate the systematic identification and management of the risk in (and imposed by the context of) the application of the technology. Residual risks must be known and communicated to the user. The AI Act also places emphasis on data governance, setting out requirements for ensuring high-quality and unbiased data sets for training AI systems. Technical documentation of the AI system must be kept and updated through its lifecycle, along with record-keeping measures, ensuring oversight and operational transparency. Users shall also be well-informed about an AI system’s capabilities and limitations, further promoting transparency. Additionally, the AI Act underlines the necessity of human oversight and inspection, allowing for human intervention, supervision and control over the system.
Havtil’s follow-up
In Havtil’s follow-up activities, it found that the industry has high ambitions and goals with regards to adopting more complex digital solutions to improve efficiency and save costs. However, companies’ approach to the development and use of AI has yet to reach maturity and even seems to be in its initial stages in several areas. In meetings with operators in 2023, Havtil found that the understanding of what encompasses AI differs across the industry; however, most differentiate between AI and physics-based models.
Most report that AI is currently not used directly as input for safety-critical systems, but is used primarily as support and expert systems for operators. Physics-based models have been used in the industry for many decades, and the techniques and processes to ensure their quality differ from those of other AI solutions. The concept of viewing the AI ecosystem as a “complex system” is increasingly utilized, emphasizing the integration of various data sets, models, control systems and their interaction. At the same time, although to a lesser degree, companies say they have the tools and methods to systematically ensure that AI-enhanced systems fully perform as intended and can communicate uncertainties to users.
It was also found that practices for governance of AI systems are less established, both for AI models and data. Several companies also assume that the data they receive from other companies or from other systems have sufficient data quality without performing further verifications.
Even though the use of industrial AI in the oil and gas sector is in its early stages, it is being employed in planning, decision support systems, and integration with other systems. Hence, for Havtil, placing prudent attention on how companies can utilize this technology in the safest possible manner is important.
In an industry with the potential for major accidents, risk must be managed in a responsible manner.
As AI is developed and utilized in operations that impact or are critical for safety, it will be important for Havtil to follow up on areas that hold particular relevance for AI safety, both from a traditional safety perspective and in light of the EU AI Act. These include risk management strategies, algorithmic transparency, governance, and validation and verification of AI.
Despite increased use of AI and automation, Havtil believes that the petroleum industry will continue to have humans in the loop for the foreseeable future. Therefore, ensuring that AI is developed and implemented with a human-centric approach, minding the automation Leftover Principle, is at the core of Havtil’s integrated human, technological and organizational perspective on AI, automation and digital safety.
How IRF perceives safety
The International Regulators’ Forum (IRF), in which offshore safety authorities from 11 countries collaborate to implement efficient supervision of safety and the working environment in petroleum activities, has defined digital technology and automated solutions as one of its three priority topics.
The prioritized topics build on common experiences that have shown that the industry, in many situations, does not pay sufficient attention to the human element when developing and adopting new technology.
Through cooperation among the 11 safety authorities, as well as industry organizations such as the International Association of Oil & Gas Producers and IADC, the aim is to contribute to an industry that places safety and the working environment high on the agenda and promotes efforts to find appropriate solutions when advanced digital technology is developed and adopted. Through active engagement with academia and industrial cooperation, necessary attention can be directed toward safety risk when developing and using AI solutions in the petroleum industry. DC
This article is based on a presentation at the 2024 IADC Advanced Rig Technology Conference, 27-28 August, Austin, Texas.