Unsynced time measurements can lead to data aggregation challenges
Basic data quality issues such as this can be addressed by deploying quality management process at the source of data collection
By Matt Isbell, Hess Corp
Oil and gas drillers have long recognized the importance of measuring, observing and recording critical loads and pressures while drilling wells. Records of drilling measurements and other information collected at the rig preserve decisions and events impacting the safety, quality, delivery and cost of each well. Today, these measurements usually come from a digital data system. Unfortunately, the accuracy and trustworthiness of the data aren’t easy to verify promptly when a safety-critical decision must be made quickly. The Operators’ Group for Data Quality (OGDQ) is addressing this issue by providing data quality guidance, such as a contract addendum for rig contractors and other vendors.
Historical Improvements in Data Capture
Drillers first began capturing data using a simple analog strip chart with critical measurements to better document the sequence of what happened and when. A drum held a chart that moved according to its clock. Traces marked every foot drilled, the pump pressure and the hookload.

Today, drilling data have been transformed. Hundreds of digital measurements are collected almost simultaneously from multiple systems. Data systems capture and display information referenced to time and measured depth, so drillers and decision-makers have detailed data to manage the drilling process. Personnel still provide input for many reports and well documents, but data systems now capture most information drillers use to manage well delivery, every foot drilled or second elapsed.
The amount of digital drilling data captured at the rig site continues to increase. As new technology is created, new uses for data and new data users emerge. Applying statistical process control techniques to digital data demonstrably increases and protects the value of a wellbore in terms of safety, quality, delivery and cost. However, in the midst of eagerness to harness the power of digital data, the old “junk in, junk out” adage still holds. The quality of the collected data will always affect the quality of the decisions made by those who use it.
Data Limitations
Drillers and support staff use drilling data displays to recognize system states. A “normal” state is defined by experience, so it takes experienced personnel to identify when processes are abnormal or potentially hazardous. Drillers must control risk and uncertainty, staying within the safety envelope of the well design. Risks that might cause a departure from the safety envelope include potential variations in downhole conditions, operational execution and many other factors. Process safety for well control is always the overriding concern, but drillers must carefully balance many well delivery considerations.
The personnel at a rig site generally understand the limitations and quality of the drilling data they collect. They have firsthand knowledge of the drilling process and involvement in moving and maintaining rig equipment. For example, drillers understand that depth measurement is the measure of the drillstring component length added to the string at surface, rather than a measurement of the actual length of the string, which varies due to temperature, material properties, friction, tension and other factors. Despite the difficulties in precisely measuring depth, this measurement is one of the two basic anchors for well data. The second anchor is the time stamp. The recorded time could be when the measurement was generated, when it was received by the data aggregator, or some other time. Well data loses its context when the time or depth reference is removed, contains error or is mismatched.
The Reuse of Data
Shale play developments are driving the reuse of drilling data because they may involve thousands of similar wells. Drillers apply continuous improvement strategies and processes to improve well design and operational execution for future wells. The users of drilling data increasingly leverage it in remote, real-time monitoring or operations centers, combining personnel and machines to advise on process safety and performance. Personnel and real-time advisory systems in operations centers, such as those performing directional drilling and geosteering, make operational decisions based on data displays for wells that are potentially located thousands of miles from the decision-makers.
Many centers use data systems constructed in layers, passing available drilling data between systems without fully considering the sources, potential errors and variabilities in the data. Data quality issues can affect the engineering logic used to identify patterns associated with normal or dysfunctional operations. Errors in time or depth data are especially detrimental because they can even shift the apparent sequence of events.
One of the first problems encountered when trying to reuse drilling data is how to understand the data origins and quality. The quality of the original data is dependent on the characteristics of sensors, signal processing, data capture systems and data aggregation systems, to name a few. Data can also be overwritten by “correction processes” that may use buffered data or other logic to fill in missing measurements or correct other errors such as a scaling factor.
Most oilfield data capture and aggregation systems were intended to collect and display drilling data at the rig site or to graphical user interfaces on the internet. Providing digital data sets to other users for analysis was done after the fact and as a single transaction referenced to time and/or the measured depth of a well.
Today, the digital data stream can be accessed in near real time and passed to multiple analytical systems. Most data systems do not provide information about the quality of the captured data. This is changing as newer digital communication protocols are created and used, but many challenges remain to measuring or establishing data quality through industry standards.
A Case Study
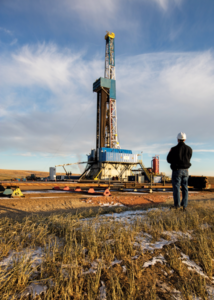
A simple example of data quality challenges comes from a study by an operator comparing three data aggregation systems, run in parallel, capturing similar data for three different sets of users. The rig contractor provided the first system, linked to the rig control system, as the original data source. This system provided digital data to the two other systems, which contributed additional measurements specific to their users.
The study found the single biggest source of error in the data was the time measurement. Each system used its own computer clock to provide an independent time measurement, which differed by 2 minutes on one system and 15 minutes on another compared with the source system. This different time and depth reference for each data set also resulted in slight differences in statistical data measures, such as the calculated averages and standard deviations over observed time intervals (Figure 1).
Even small differences create problems in determining the sequence of events when comparing one data set with another. If a user compares one system that is used for monitoring well control pressure with another system on a nearby display that shows mud circulation, the user can’t know which set of events to believe to determine corrective action.
Fortunately, when a specific data quality problem has been identified, countermeasures can be applied. In the case involving mismatched computer clocks, all three systems could be synced to a network clock. In the absence of a common network, hardware can be introduced that uses a broadcast national atomic clock or the global positioning system satellite clock. New communication protocols can also be applied to correct this issue, which can track original time stamps and other successive time stamps when the measurement is passed between systems. Operators will need to address these issues through rig contractor and vendor systems.
Addressing Data Quality as an Industry
Data quality is difficult to improve after collection; the best practice is to manage it from its source. This requires applying a quality management system to control and track the data measurement systems, aggregation systems and data transformations contributing to the final data in its end-use state. A simple data quality management process builds a data register of measurements and traces the data flow from the sensor to the end measurement or vice versa. This method tracks the quality inherent to the data. Ideally, the end user defines the data quality requirements based on the intended use of the data.
Operators who recognized the need to advocate for improvements in data quality formed the OGDQ in 2014. The group initially focused on basic data quality definitions and created rig contract language to manage data quality elements. It also publishes data quality examples and potential improvements in processes and tools. The OGDQ creates industry awareness of data quality to contribute to safer and more productive operations.
The OGDQ recommends that data users take responsibility for verifying and managing the quality of their data. However, data quality must be controlled by each entity that touches the data. Owners of existing data systems should publish data quality measures so users understand the limitations of the data employed by their systems. Data providers must have systematic methods and processes for calibrating, verifying and validating sensor measurements. Data aggregation should be tied to multiple references, such as a time and measured depth, using a defined method to track when changes or corrections are applied.
The digital tools and resources available to drillers to use in addition to their experience will continue to improve and gain complexity. The OGDQ seeks a future where drillers have the trust to make decisions from accurate drilling data through competent data quality management. DC