Automated advisory system aims to provide accurate predictions for optimized fluids management
Algorithms work within a self-learning framework to detect fluid treatment decision points, recommend actions for field engineers
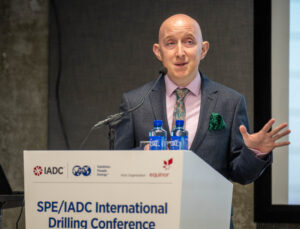
By Stephen Whitfield, Senior Editor
The management of drilling fluid properties has long been neglected as a method of increasing drilling performance, partly due to the complexity involved. Even best practices and engineering manuals give general concentrations to aim for or to maintain, rather than prescribing a precise course of action. Furthermore, due to the uncertainties associated with modifying the fluid system, it can be nearly impossible to accurately predict the result of an adjustment.
This situation can lead to several problems, said John Whyte, Domain Champion at SLB Cambridge Research. Due to fear of over-treatment, for example, engineers often wait lengthy periods of time before making a treatment.
This caution, Mr Whyte said, can lead to the fluid being suboptimal for longer than required. Additionally, the treatments themselves are often just educated guesses, as fluids engineers have no means to calculate the exact properties that will result after a treatment is made.
“There’s a lot of complexity in the interactions between all the components in drilling fluid,” he explained. “We’re incorporating all kinds of solids of different levels of reactivity while we’re drilling, along with things like brine flows and so on that can react with the fluid in ways that we wouldn’t want. These can all be very difficult to deal with, and the speed of detection of any deviations is one of the most important things.”
At the 2025 SPE/IADC International Drilling Conference in Stavanger, Norway, on 4 March, Mr Whyte outlined the development and testing of a quantitative fluid advisor designed to determine the exact treatments that a drilling fluid might require and accurately predict the state of the fluid after treatment.
The software program is made up of interconnected algorithms that work together to give quantitative recommendations of fluid treatment and management. It can identify situations where treatments should be made, calculate the treatment required to achieve a given objective (for example, returning a property to its target value) and decide on the value of that decision (for example, predicting if the improvement in fluid quality is sufficient to justify the time and cost of a given treatment).
The advisor is designed to augment decision making by the fluids engineers in the field, rather than replace them entirely. It analyzes field data automatically collected from sensors installed along the drillstring in order to make treatment recommendations, which are based on current drilling conditions and the position of the fluid within a given specification window. It also identifies the engineer’s decision points in a standard operation.
When a situation is identified, the advisor provides treatment options and the recommended outcome of those options. A quantitative module within the software then calculates the amount of treatment required to achieve the recommended outcome, as well as a prediction of the time until a negative effect is likely to occur without treatment.
“This system is a mix of different types of advice,” Mr Whyte said. “One on hand, there’s the qualitative advice, the plain English description. Here’s what we’re recommending and here’s our explanation as to why we’re recommending it. Then there’s the quantitative solution. Here are the number of kilograms of a given product that will result in these subsequent properties. It’s important to us that the rig crews are still in control of this process. Ultimately, we’re providing options, and they have the final say on the treatment.”
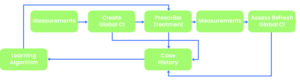
After a treatment is made, the advisor automatically assesses all data channels to detect changes in relevant fluid properties and identify changes. Then, once an equilibrium state is reached, it will compare the actual property changes in the fluid against predicted changes.
Results are also added to a library of case histories. This helps to create new recommendations for future wells by modifying the treatment model’s suggestions. Effectively, the advisor is a self-learning system in which every treatment becomes its own data point.
Mr Whyte noted that users can also input their perceived level of experience into the software, which will influence what level of advice is offered. For more experienced operators, the advisor concentrates on what it deems as critical interventions. Other instances are de-prioritized, with the system assuming that an experienced user could spot those non-critical situations on their own.
In order to comprehensively assess the overall fluid quality and the effects of treatment on the fluid, the advisor also constructs a condition index (CI) based on fluid measurements taken while drilling. The CI is designed to aid the fluids engineer in the decision-making process by enabling a greater understanding of the status of the fluid before and after treatment.
“By using this index, we can have fully autonomous decision making. Not only can we identify something that needs to happen, we can calculate what should be done, and the system can actually decide for itself whether or not to pursue that course of action. If you want to, say, have a maximum cost per meter for a treatment, the advisor can take that into account and recommend different treatments based on that,” Mr Whyte said.
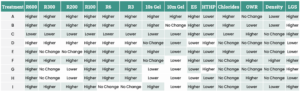
Field test results
SLB Cambridge conducted field testing of the advisor on a 17.5-in. section of a development well in the UK North Sea, located in a water depth of 338 ft. The fluid used to drill the section was a low-toxicity oil-based mud.
Remote personnel monitored the system from SLB’s Performance Live Centre in Aberdeen, Scotland, and passed on advice generated by the advisor to the fluids engineers at the wellsite. All treatments added were modeled for their predicted effect, and each prediction was relayed to the field engineers, who were also able to request predictions on speculative treatments.
SLB had five objectives for the test:
- to deploy the advisor during an operation without gaps in service;
- to provide fluids treatment advice to the fluids engineers based on field data ingested by the system;
- to model expected system reaction and compare the actual result of treatments made during drilling;
- to test the system’s ability to identify a treatment automatically, define an appropriate treatment, and determine if that treatment is advisable without human input; and
- to provide advice on how to treat the fluid.
In the field test, the advisor was deployed successfully with no gaps in service. It was also run in near-real time – updated as soon as new data became available, with no significant delay in relaying advice to engineers.
SLB evaluated the success of each prediction generated by the advisor by comparing predicted changes in fluid properties with actual changes after treatment. For instance, if the advisor predicted an increase in chlorides level from a treatment, did the chlorides actually increase when those treatments were applied?
Testing results showed a good level of prediction accuracy, with 94 out of 132 property changes matching the actual property changes post-treatment (see Table 1). Rheological properties were often within experimental error – both the magnitude of the predicted values and the relative values intra-prediction matched those measured by the offshore engineers.
The mean absolute percentage error (MAPE) of each prediction was also calculated in order to account for any discrepancies in the magnitudes of the property values between treatments. While Mr Whyte acknowledged that there is no consensus as to the optimal measurement error for a fluid property check, SLB established a 10% KPI as the benchmark, believing it was reasonable based on experimental error accepted on QC laboratory testing across the company.
Among the nine treatments recommended by the advisor during the test, the MAPE ranged from 2.8% to 9.0%. The average error across the test was 5.4%.
Mr Whyte noted that the field engineers agreed with 100% of the qualitative advice given by the advisor. By the end of the test, engineers were interacting with the fluid system differently – they were asking the system what would happen in a given scenario and adjusting their plans accordingly. This, he said, was the most critical element of the field test.
“That was a really interesting emerging behavior. They started asking the system, are there better ways of doing a treatment? Can we combine lots of treatments into one? That was really good to see. The advisor is designed to provide all the possible flags and the quantitative advice at the earliest possible opportunity. Offshore engineers are slightly cautious and want more data points before committing even when the software is proving correct, but if the system says something, you can trust it straight away.”
Mr Whyte added that more testing will be needed before the advisor can be implemented for widespread commercial release. The advisor was developed and field-tested using SLB fluid systems, so additional algorithms will need to be developed that can take into account non-SLB products.
“We’re aiming for this to be product agnostic,” he said. “From a scientific point of view, there’s no reason why that shouldn’t be possible.” DC
For more information, please see SPE/IADC 223676, “A Quantitative Drilling Fluids Advisor.”