Drilling fluid digital twins developed to monitor rheological properties, cuttings transport functionality
Predictive models were built by collecting extensive lab data and CFD simulation results that were fed into automated machine learning algorithms
By Stephen Whitfield, Associate Editor
Drilling fluids play an assortment of critical roles in the success of drilling operations, like transporting cuttings, cooling the drill bit and suspending the cuttings when circulation stops. Many drilling problems, including circulation loss and stuck pipe, also stem from drilling fluids. That’s why developing accurate digital twins for drilling fluids could be critical in anticipating and preventing these problems, by enabling better monitoring of downhole fluid properties, such as rheology and density, and functionalities like borehole stability.
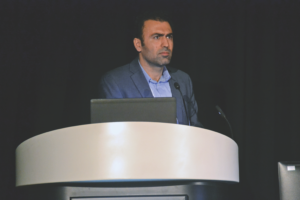
Speaking at the 2020 IADC/SPE International Drilling Conference in March, Mehrdad Shirangi, Staff Data Scientist at Baker Hughes, outlined the company’s development of digital twins for monitoring drilling fluid properties and associated wellbore phenomena during drilling operations. The goal was to be able to model accurate properties of downhole fluids in high-pressure, high-temperature (HPHT) conditions, allowing for more efficient and safer operations.
Digital twins are built through a three-step closed-loop process. The first step is the development of a conceptual design that considers value-adding objectives, historical data and real-time data. The second step is the implementation of analytics and the eventual deployment of the digital twin, and the third step is the operation. This process is repeated periodically, with learnings from the operation feeding back into the system, enabling design adjustments and enhancements.
Mr Shirangi said the ability to learn from both historical and real-time data is an essential property of digital twins, because the learnings from a model of one asset can be translated into different operations around the world.
“When we talk about digital twins, the concept is usually thought of as something like a cloning device, where we combine the software and the physics that we have. From a data science perspective, however, a digital twin is all about database management. You’re creating a database that’s continuously updated. You need to understand these features that you want to utilize in the digital twins,” Mr Shirangi said.
The company utilized automated machine learning algorithms to build predictive models that were deployed in the planning of a drilling fluid system. Prior to building its own model, Baker Hughes investigated two elements of a conceptual drilling fluid digital twin to see if they could be accelerated through the use of machine learning platforms:
- Real-time prediction of the rheological properties of downhole fluids; and
- Local velocity estimation above the cuttings bed.
A machine learning-based predictive model could also be used to improve hole-cleaning calculations, Mr Shirangi said. Hole cleaning is one of the most important functions of drilling fluids, as inadequate hole cleaning can lead to excessive development of the cuttings bed, spawning costly drilling challenges, such as excessive torque and drag on the drill string. Typically, these hole-cleaning calculations are considered at the well planning stage, but during drilling operations, certain parameters may deviate from the original plan. By using a predictive model, new calculations could be done at the rig site in a matter of seconds.
An important parameter for correctly predicting hole-cleaning conditions during drilling is the local velocity above the cuttings bed as a function of operating conditions. For the study, Baker Hughes developed computational flow dynamics (CFD) simulation models and applied two-dimensional CFD simulations for a range of parameters like cuttings bed heights, rotation, wellbore size and eccentricity for a set of field-relevant cases. Those simulations helped determine the average fluid velocities above the cuttings bed.
Mr Shirangi said the digital twin allowed for a more accurate calculation of cuttings bed height along the wellbore, but the parameters studied had a complex relationship with each other. When the cuttings bed is far from the wellbore wall, rotational speed does not significantly affect the average fluid velocity. When the cuttings bed height reaches 60 mm, average velocities become more sensitive to rotational speed.
In a case with no rotation, fluid velocity decreases as the cuttings bed height increases, primarily because of the formation of a low-velocity region between the cuttings bed and the lower side of the drill string. Mr Shirangi said a single parameter can have a noticeable impact on accuracy.
“If you change a number of variables, it affects the accuracy quite a lot. If we change the maximum value of one particular variable, it affects the cross-validation accuracy of the model. This is a variable optimization problem. You cannot optimize one parameter at a time,” he said.
The Baker Hughes team also examined the real-time determination and monitoring of downhole fluid properties, which Mr Shirangi said was important for safe drilling operations. Their goal was to utilize surface measurements at API standard conditions and data from compositional analysis to estimate the rheological properties of the drilling fluid in downhole conditions.
Typically, the properties that fluids exhibit when put into downhole HPHT conditions are determined through lab experiments that take between one and three days. Like with hole cleaning, the digital twin could allow operators to determine these properties at the rig site in seconds.
Future work will involve the building of digital twins for other functionalities of drilling fluids, like ROP optimization and transmitting hydraulic horsepower to the bit. A closed-loop optimization framework could then be developed from this, recommending optimal operational parameters based on real-time drilling data. DC