BJ Energy Solutions enters agreement for emissions-reducing TITAN technology
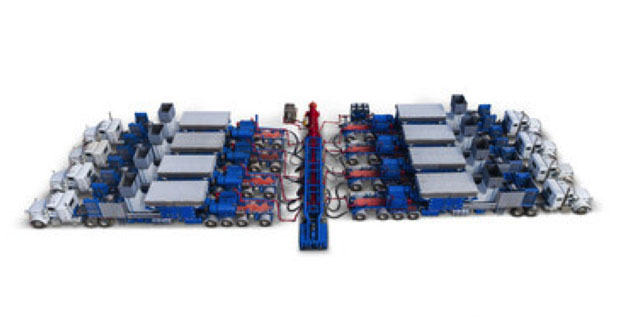
BJ Energy Solutions has entered into a three-year pressure pumping services agreement with an unnamed multinational oil company for its TITAN next-generation technology. The natural gas-powered TITAN fleet will be deployed for the company in its Duvernay resource play near Fox Creek, Alberta.
“We are pleased to have the opportunity to expand upon our existing partnership with a like-minded customer focused on responsible and sustainable production of energy. Utilizing the TITAN technology, we look to build upon the exceptional operational efficiencies achieved to date, with the added benefits of lower emissions, diesel elimination, and lowering the overall carbon footprint of the completion operation in the Duvernay,” said Warren Zemlak, CEO, BJ Energy Solutions.
At the core of the TITAN technology is a direct drive 5,000-hp natural gas-powered turbine driven pump. Fueled by natural gas, the TITAN supports the reduction of greenhouse gas emissions, reduced costs, improved mobility, and reliable operations while meeting the most stringent noise reduction requirements across North America.
“We continue to make great strides with TITAN. The first 40,000 horsepower fleet has now been in operation for over a year in the high-pressure Haynesville Basin, completing over 1,600 stages and 5,000 pumping hours, including recording 500 pumping hours in March 2022. The TITAN Technology is earning its place as one of the premier Next Generation Fracturing Solutions in the market and executing our sixth long term contract is yet one more indicator that its value is being recognized,” Mr Zemlak said.
BJ Energy expects to have four TITAN Fleets in operation by mid-year 2022, with further expansion planned in 2022 and 2023.